Big data vs small data: In the realm of data analysis, two terms often come up: big data and small data. Both are valuable in their own right, but they serve different purposes and are managed differently. Let’s delve into the differences between these two types of data.
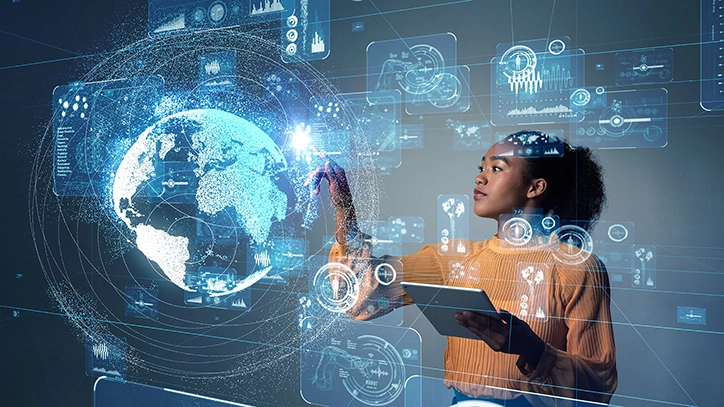
Big Data: Big data refers to extremely large datasets that may be analyzed computationally to reveal patterns, trends, and associations, especially relating to human behavior and interactions. Big data is characterized by its volume, velocity, and variety, often referred to as the three Vs.
Volume: Big data is, as the name suggests, massive in volume. It encompasses huge amounts of data, often ranging from terabytes to petabytes.
Velocity: Another key aspect of big data is its velocity, or the speed at which data is generated and processed. With the advent of social media and the Internet of Things (IoT), data is generated at an unprecedented rate.
Variety: Big data comes in various forms, including structured data (like databases), unstructured data (like text), and semi-structured data (like XML files). Managing this variety of data requires specialized tools and techniques.
Small Data: On the other hand, small data refers to datasets that are small enough for human comprehension. While there is no strict definition of small data, it generally refers to datasets that are manageable in size and can be analyzed using traditional data analysis tools.
Volume: Small data is characterized by its relatively small volume. It can be as simple as a spreadsheet or a few hundred customer records.
Velocity: Unlike big data, small data does not have the same velocity. It is generated at a slower pace and is often more static in nature.
Variety: Small data is usually well-structured and homogeneous. It is typically easier to organize and analyze compared to big data.
Key Differences:
- Size: The primary difference between big data and small data is their size. Big data refers to large datasets that require specialized tools and techniques for analysis, while small data is manageable by traditional data analysis methods.
- Complexity: Big data is often more complex than small data due to its volume, velocity, and variety. Small data, being more limited in size and scope, is generally less complex.
- Use Cases: Big data is often used for tasks like predictive analytics, machine learning, and trend analysis, where large amounts of data are required. Small data, on the other hand, is more suitable for tasks like business intelligence, customer relationship management, and market research.
In conclusion, while big data and small data serve different purposes and require different approaches, both are valuable sources of information that can provide valuable insights when analyzed effectively.
Understanding the differences between these two types of data can help organizations make better-informed decisions based on their data needs and capabilities.